Artificial intelligence technologies like facial recognition, machine learning, and computer vision are driving some of the biggest innovations in the tech world today. But these AI tools have a major diversity problem.
Facial recognition programs consistently misidentify People of Color (POC). Earlier this year, Robert Julian-Borchak Williams, who is Black, was arrested for a crime he didn’t commit based on a failed match from a facial recognition database. Self-driving cars reportedly have trouble identifying darker-skinned pedestrians, putting them at risk of fatal injury. And bias is consistently baked into the artificial intelligence systems that increasingly make staffing, medical and lending decisions.
Fixing AI’s diversity problem is hard. In many cases, the problem comes down to data. Modern AI systems are often trained using copious amounts of data. If their underlying training data isn’t diverse, than the systems can’t be, either. This is especially problematic for fields like facial recognition. Most facial recognition datasets focus on white males. According to the New York Times, one popular database is 75% male, and 80% white.
This makeup likely reflects the biases of the databases’ creators. But it also reflects the fact that these databases are often trained on public images from the Internet, where, as Wired puts it “content skews white, male and Western”.
One fix would be to ask lots of POC to contribute their likenesses to facial recognition databases. But after events like Williams’ false arrest, it’s likely that many would — understandably — be reluctant to do so. According to a study by Pew, 60% of White Americans trust law enforcement to use facial recognition responsibly. For Black Americans, that number drops to 43%, an 18% difference.
This creates a chicken and egg problem. Facial recognition will fail on diversity until it has better training data depicting POC. But at the moment, this training data largely doesn’t exist. And given that producing it would require asking thousands of POC to include their faces in training sets and potentially put their identities at risk, sourcing enough training data to fix facial recognition’s diversity problem is a daunting challenge.
A new startup, Generated Media, thinks they have a solution: create artificial People of Color. The company has built a massive dataset of photos of artificial people of all ages, genders, and racial and ethnic backgrounds. Its clients are using the photos to better train facial recognition platforms and other AI systems to recognize diverse faces. The company’s artificial people are uncannily real. Case in point: the smiling man at the top of this article. He doesn’t actually exist — he was dreamed up by Generated Media’s tech.
I talked with Alena Pashpekina, Director of Partnerships at Generated Media, about their technology, the importance of supporting diverse creators, and the emerging world of synthetic content.
According to Pashpekina, Generated Media’s images are created using Generative Adversarial Networks (GANs), a relatively new technique in the AI world, which was only fully demonstrated in 2017. GANs work by taking two neural networks, and having them square off against each other in a high-tech game of cat and mouse.
To create artificial people, a GANs system starts with a huge set of images of real people (on which more below). A neural network (called the “generative” network) looks at these, and learns what a real human face looks like. It then starts to generate its own faces from scratch, based on what it’s learned. A second neural network (called the “discriminative” network) looks at its output, as well as images of real faces, and tries to guess which are real, and which are fake.
At first, the generative network is terrible at its job. Its faces look like some horrifying, melted version of a human, with eyes in the wrong places, weird blobs instead of a nose, and the like. The discriminative network has no trouble labeling these as fakes. Over time, though, the generative network learns from its mistakes, and from the feedback that the discriminative network provides. It gets better and better at producing convincing fake faces.
The catch is that the discriminative network is also learning. With training, it comes to know when it’s successfully called out the discriminative network for producing a fake, and when it’s failed and let a fake slip past. As the networks duke it out and try to fool each other (this is the “adversarial” part of the acronym GANs), each gets better at its job.
At the end of a certain number of training cycles, the discriminative network departs, like a coach who has taught a player all that they know, and is no longer needed. The generative network is now on its own. It can happily continue to churn out convincing fake faces. The only entity it has left to fool is us — its human users.
A common GANs system for creating fake faces is called StyleGAN. It’s the system behind the popular website ThisPersonDoesNotExist.com, which allows users to conjure up fake faces in their browser. According to Pashpekina, this is the same system that Generated Media uses to create its proprietary artificial people. “Initial training happens with a tuned StyleGAN model with proprietary input data we create,” Pashpekina told me. “After images are produced, we use further machine learning to separate out the background layer, categorize the outputs, make sure all metadata is valid, and provide a strong level of quality control.”
That last part is crucial, because the output of even a well-trained GAN can range from surprisingly convincing to disturbingly bizarre. Faces sometimes emerge from a generative network with eyes in the middle of their foreheads, or hair that randomly stops in a sharp edge. Other times they’re adorned with the computer’s strange guesses at what human jewelry, glasses or headwear look like. GANs are so bad at creating fake jewelry that looking for asymmetries like “different earrings in the left and right ear” is one way that researchers say people can tell an artificial face from a real one.
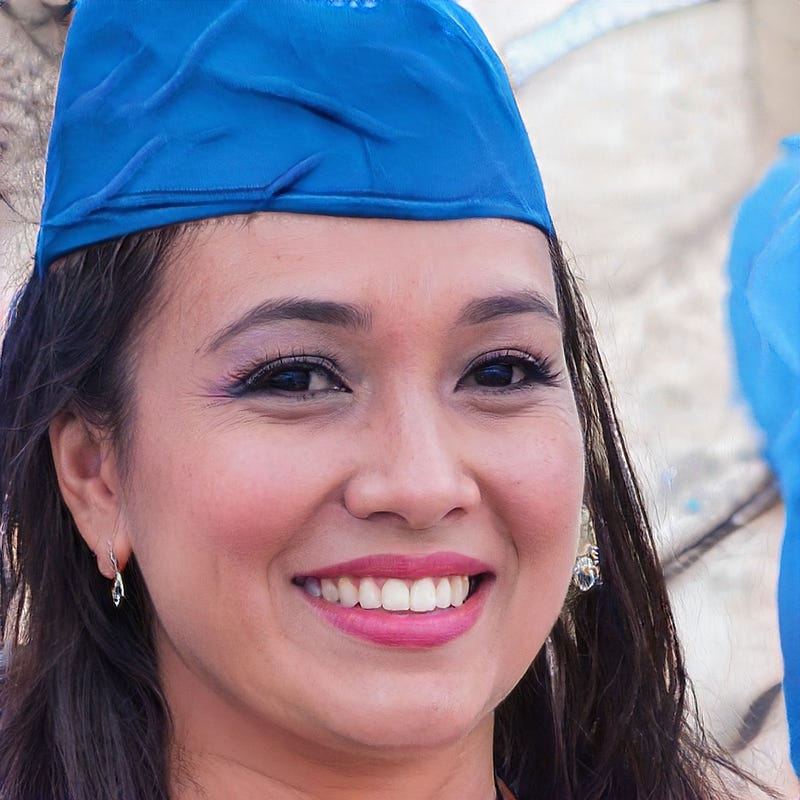
Generated Media’s team curates the output of their networks, removing these obvious fakes and making other subtle tweaks to ensure their artificial people look as real as possible. The team also assigns metadata to each image, describing the artificial person’s age, gender, race, head position and more.
In a process that feels more than a little Orwellian, visitors to the company’s website Generated.photos can use a series of drop-downs to enter a specific Ethnicity (White, Black, Latino, or Asian), Age, Gender, Emotion, Eye color, and Head position. They’re then presented with hundreds of images of artificial people meeting their exact specifications. Each face can be placed on a custom-colored background. In total, more than 915,000 artificial faces are available.
In addition to offering a-la-carte faces, Generated Media also offers large datasets of artificial faces. Unlike the racially-skewed datasets of real faces underlying most existing AI systems, the company’s datasets are explicitly designed to include an even racial balance (25% each of White, Black, Latino, and Asian faces), as well as a 50/50 gender balance. According to Pashpekina, “This is not something that ‘just happens’. You have to make special processes that allow for generating images by specific attributes” like race and gender. The company sells access to these datasets, but also provides free access to many academic researchers.
Generated Media’s clients use these datasets for a variety of purposes. These include training facial recognition platforms to better recognize diverse faces. According to Pashpekina, Generated Media is “able to make large datasets that have less racial bias than almost any dataset out there.” The racially diverse datasets of artificial people generated by their GANs technology, Pashpekina told me, “help reduce racial bias in facial recognition systems.”
Training facial recognition software using the output of another AI system feels like it shouldn’t work. Like making a photocopy of a photocopy, you’d expect a major loss in quality. But using synthetic data is already a standard practice in AI. In fact, GANs were originally created to generate synthetic data for use in training other AI systems. And according to an IBM researcher with whom I spoke, generating artificial faces is a viable way to train a facial recognition or other machine learning system, even if they’re not quite as good as real faces.
Generated Media’s datasets have another advantage over existing options, too. As the New York Times describes, many datasets used to train GANs systems today are sourced from images found on the public Internet. According to Nancy Wolff, a copyright attorney, this creates all kinds of thorny legal and ethical challenges.
Many of these relate to ownership and consent. If a company uses a GANs system to create artificial faces based on the faces of thousands of real people, do those real people need to give their consent first? What if the photos of their faces are covered by copyrights — are the artificial faces “derivative works”? If so, would the owner of the GANs system need a copyright license for each training image they used? Would privacy laws like California’s CCPA apply?
According to Wolff, these questions (many of which have yet to be answered by courts) create “real liability” for companies that build GANs and other AI tech using public images. Issues of consent are especially acute for POC, many of whom would likely object to their faces being used in a police database or facial recognition platform without their approval.
Generated Media sidesteps this problem by creating all their training data themselves. Their GANs platform is trained on over 92,000 face images of actual people, who sit for portrait sessions at Generated Media’s in-house studio. The company hires “diverse models to pose for photos that we can use for training data”, Pashpekina told me. All models sign consent forms, explicitly allowing their faces to be used for training Generated Media’s GANs platform, as well as other machine learning systems. They’re also compensated for their time, just like any other paid model.
This hybrid model–photographing real POC, but using their likenesses to generate faces of people that don’t actually exist–is a compelling way to help address AI’s diversity problem. The company’s artificial people are essentially complex, computer-imagined amalgams of the faces of hundreds of real, diverse people. That makes them a powerful tool for improving the diversity of facial recognition systems and other AI platforms, by providing better, more balanced training data.
Yet at the same time, the fact that their people are artifical protects the identities and privacy of actual POC. Even if Generated Media’s artificial faces were used to train sensitive systems like a a police agency’s facial recognition database, the police agency wouldn’t be able to see the faces of the real POC on which those artificial faces were based. They could improve the accuracy of their system in recognizing the faces of POC, without putting the identities of real POC at risk, or forcing them to contribute their likenesses to a database without their consent.
It’s a model that Generated Media hopes to use in other places where customers need sensitive data. According to Pashpekina, journalists have used the company’s images to protect the identities of sources, academics have used them in bias research, and clinicians have used them for dementia testing. The company also offers the images as stock photos, for users who “don’t have the time, ability, or budget to go through the photography process” (or can’t because of Covid-19), but still want to use diverse faces on a product label or in an advertising campaign.
When any individual or company can create an artificial image and pose as a real POC (or imply their endorsement), this creates important questions of veracity and representation. Should anyone be permitted to leverage these images for any purpose? Would it be right for groups to use images of Black or Brown people–and potentially profit from those uses–if the group itself is not diverse? Where is the line between inclusion and misrepresentation? All of these are complex questions which will need to be more fully explored as synthetic image technology continues to mature.
More pragmatically, selling artificial images as stock photos risks taking work away from real POC photographers and models. Pashpekina acknowledges this, but says that “photography is a very large market, and we don’t see this as a zero-sum game. There will always be situations where it makes more sense to hire a model, a photographer, and scout a location.” Generated Media also encourages people to buy diverse stock photos from POC creators, noting that “we also do this ourselves…hiring diverse models to pose for photos that we can use for training data” (I recommend Mocha Stock, PICHA, and Nappy.co if you’re looking for photos of real, diverse people from POC-owned agencies).
Artificial people aren’t perfect. Ideally, AI training datasets would include a balanced set of photos of diverse, real people (and would do so without putting those people at risk). But until that happens, creating artificial POC is a fast way to add much-needed diversity to existing AI systems, while minimizing the risk to individuals, and protecting their rights. Generated Media’s people may be artificial. But the potential benefits they offer in terms of inclusion, AI accuracy, safety, and privacy are very real.